Harnessing Artificial Intelligence (AI) in the fight against COVID-19
AI assisted COVID-19 diagnostic and prognostic tool could improve resource allocation and patient outcomes
An Artificial Intelligence (AI) tool, combining chest imaging data with laboratory and clinical data, is being developed by members of the Cambridge Mathematical Imaging in Healthcare Centre (CMIH) to support rapid diagnosis and triaging of patients with COVID-19 in the UK. The multidisciplinary team, led by Professors Carola-Bibiane Schönlieb and Evis Sala, brings together expertise in AI for imaging from CMIH with expertise in radiology and clinical applications from Addenbrooke's and Papworth Hospitals to develop a prediction model that can rapidly and reliably diagnose and suggest a prognosis to the clinician.
Reverse-transcription polymerase chain reaction (RT-PCR) tests are currently the key approach used for diagnosing COVID-19, but the high false-negative rates and low sensitivity of the lab tests can lead to late diagnosis and treatment. Whilst chest x-rays and CT scans provide valuable diagnostic and monitoring information that can complement laboratory and clinical data, expertise is required to interpret the severity of the findings, typically by radiologists, who are inundated with COVID-19 patients. Fast and accurate diagnosis of patients together with the rapid determination of whether a patient is likely to recover, require intensive care unit (ICU) admission or intensive ventilation is key to triaging resources and to improving patient outcomes.
AI offers huge potential to support agile clinical decision making, ensuring patients receive the most appropriate support and leading to better patient outcomes.
Rigorous mathematical models play a key role in mitigating bias and improving efficacy of the prediction model as they follow universal rules with mathematical guarantees.
Recent studies have shown that leveraging AI could have a real impact on the management of patients with COVID-19. AI tools such as Deep Learning (DL) can offer automated image interpretation and integration with clinical data, leading to improved diagnosis and patient triage, better treatment response assessment and outcome prediction. However, large amounts of data and computing power are required to train and optimise a DL model and data availability is one of the key bottlenecks in developing systems. Coupled with this, the lack of standardisation of datasets makes it challenging to reuse existing AI tools in a country that is different to the one that it has been trained for, as its predictions are inherently biased. The data that has been used to train models also needs to be systematically shared.
Addressing these challenges, the Cambridge team is working to develop a flexible, open-source AI tool that could be used by hospitals worldwide. Drawing on a longstanding history of global research collaboration and expertise in data governance, the team is amassing datasets from Austria, China, Italy and the UK to initiate their work. Data scientists and clinicians are working in close collaboration, following standard protocols to identify bias during development.
Using the latest Deep Learning approaches in conjunction with mathematical and statistical analysis methods, the new tool will be accompanied by a comprehensive algorithmic strategy that will allow fine-tuning to datasets with different characteristics and implementation in different countries.
The core project team is comprised of expert data scientists and clinicians drawn from across Cambridge and is led by Professors Carola-Bibiane Schönlieb, Director of the Centre for Mathematical Imaging in Healthcare and Evis Sala, Professor of Oncological Imaging, University of Cambridge & Honorary Consultant Radiologist, Addenbrooke's Hospital, Cambridge University Hospitals NHS Foundation Trust. This team is supported by an AI and image analysis team, drawn from subject experts across Cambridge and around the world, a clinical team comprised of colleagues from hospitals in Cambridge, London and Vienna, and a support team based in the Faculty of Mathematics. Partner institutions include hospitals in Wuhan, China; Milan, Italy; and Madrid, Spain, and universities in Manchester, Vienna and London.
The University of Cambridge has an impressive record of achievement in multidisciplinary research and innovation. The CMIH is a collaboration between mathematics, engineering, physics, and biomedical scientists and clinicians and is one of 5 centres to receive investment from the Engineering and Physical Sciences Research Council (EPSRC). A key aim of this partnership is the delivery of high quality, multidisciplinary research that will help overcome some of the big challenges facing the NHS.
Philanthropy plays a vital role in supporting research in the fight against COVID-19. If you are interested in making a gift towards this project please contact
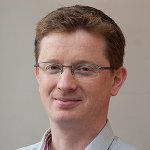
Glen Whitehead
glen.whitehead@admin.cam.ac.uk
+44 (0)1223 330112 or mobile: +44 (0)7711 500332
Make a gift now
Make a gift now to support Cambridge COVID–19 Research Fund by credit or debit card, or set up a direct debit:
Or, find out about other ways to give.
Glossary of terms
Clinical data: including age, sex, temperature, time since symptoms start and PO2 (Partial Pressure of Oxygen). PO2 reflects the amount of oxygen gas dissolved in the blood. It primarily measures the effectiveness of the lungs in pulling oxygen into the bloodstream from the atmosphere.
RT-PCR test: a test that can extract, amplify and detect the virus’ genetic code.
False-negative: when a test improperly indicates no presence of a condition.
Open-source: software for which the original source code is made freely available and may be studied, redistributed and modified.
Inherent bias: the effect of underlying factors or assumptions that skew viewpoints of a subject under discussion.
Deep learning: machine learning based on artificial neural networks that are trained to process data and create patterns for use in decision making.